Welcome to CISA — Opening Remarks and Overview of NIST
Dr. Christopher Holloway, NIST

Dr. Christopher Holloway is a NIST Technical Fellow and a Fellow of the IEEE and has been at NIST for over 25 years. He is also on the Graduate Faculty at the University of Colorado at Boulder. His is an expert in electromagnetic theory and metrology, quantum-optics, Rydberg-atom systems, and atom-based sensors. He has a publication h-index of 64 with over 300 technical publications (including 152 refereed journal papers and 133 conference papers) and has over 15,400 citations of his papers. He also has 10 patents in various fields in engineering and physics. He is the Project Leader for the Rydberg-Atom-Sensor Project and is the Group Leader for the Electromagnetic Fields Group.
Reference Waveforms and Reference Channels for mmWave Wireless Device Testing
Dr. Kate Remley, NIST
The use of over-the-air (OTA) test is a necessity for characterizing mobile microwave and millimeter-wave wireless devices with antennas that are integrated into transmitters and receivers. Lab-based OTA test allows repeatable testing in realistic conditions, but isolating and characterizing potential distortion-inducing effects in the test set-up is critical, especially at millimeter-wave frequencies. Recent work at NIST in this area will be presented including the use of synthetic-aperture channel characterization for testing directional wireless internet-of-things devices in realistic, repeatable conditions.
Kate A. Remley (S’92-M’99-SM’06-F’13) was born in Ann Arbor, MI. She received the Ph.D. degree in Electrical and Computer Engineering from Oregon State University, Corvallis, in 1999. From 1983 to 1992, she was a Broadcast Engineer in Eugene, OR, serving as Chief Engineer of an AM/FM broadcast station from 1989-1991. In 1999, she joined the RF Technology Division of the National Institute of Standards and Technology (NIST), Boulder, CO, as an Electronics Engineer. She was the Leader of the Metrology for Wireless Systems Project at NIST, where her research activities included development of calibrated measurements for microwave and millimeter-wave wireless systems and standardized over-the-air test methods for the wireless industry. She chaired the working group that published the IEEE 1765-2022 Recommended Practice on EVM Measurement and Uncertainty Evaluation from 2016 to 2022. In July 2022, she retired from NIST. Dr. Remley is a Fellow of the IEEE and was the recipient of the Department of Commerce Bronze and Silver Medals, an ARFTG Best Paper Award, the NIST Schlichter Award, and is a member of the Oregon State University Academy of Distinguished Engineers. She was the Chair of the MTT-11 Technical Committee on Microwave Measurements (2008-2010), the Editor-in-Chief of IEEE Microwave Magazine (2009-2011), and Chair of the MTT Fellow Evaluating Committee (2017-2018). She was a Distinguished Lecturer for the IEEE Electromagnetic Compatibility Society (2016-2017).
Solving Inverse Problems with Generative Priors: From Low-rank to Diffusion Models
Prof. Yuejie Chi, Carnegie Mellon University
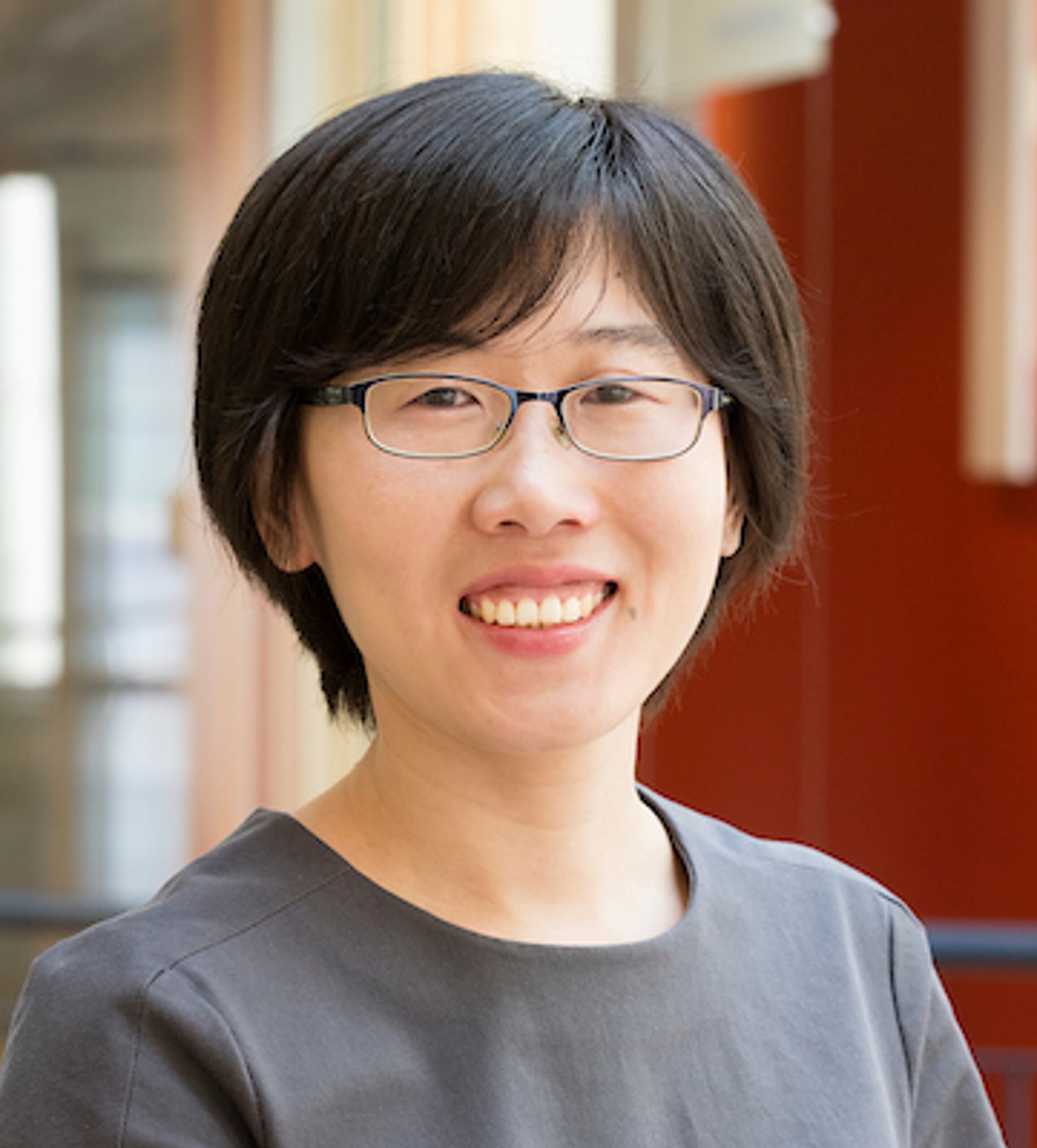
Generative priors are effective countermeasures to combat the curse of dimensionality, and enable efficient learning and inversion that otherwise are ill-posed, in data science. This talk begins with the classical low-rank prior, and introduces scaled gradient descent (ScaledGD), a simple iterative approach to directly recover the low-rank factors for a wide range of matrix and tensor estimation tasks. ScaledGD provably converges linearly at a constant rate independent of the condition number at near-optimal sample complexities, while maintaining the low per-iteration cost of vanilla gradient descent, even when the rank is overspecified and the initialization is random. Going beyond low rank, the talk discusses diffusion models as an expressive data prior in inverse problems, and introduces a plug-and-play method (Diffusion PnP) that alternatively calls two samplers, a data-dependent denoising diffusion sampler based solely on the score functions of data, and a data-independent sampler solely based on the forward model. Performance guarantees and numerical examples will be demonstrated to illustrate the promise.
Dr. Yuejie Chi is the Sense of Wonder Group Endowed Professor of Electrical and Computer Engineering in AI Systems at Carnegie Mellon University, with courtesy appointments in the Machine Learning department and CyLab. She received her Ph.D. and M.A. from Princeton University, and B. Eng. (Hon.) from Tsinghua University, all in Electrical Engineering. Her research interests lie in the theoretical and algorithmic foundations of data science, signal processing, machine learning and inverse problems, with applications in sensing, imaging, decision making, and societal systems, broadly defined. Among others, Dr. Chi received the Presidential Early Career Award for Scientists and Engineers (PECASE) and the inaugural IEEE Signal Processing Society Early Career Technical Achievement Award for contributions to high-dimensional structured signal processing. She is an IEEE Fellow (Class of 2023) for contributions to statistical signal processing with low-dimensional structures.
Capturing our Dynamic Galactic Black Hole with Computational Imaging
Prof. Katie Bouman, CalTech

At the heart of our Milky Way galaxy lies a supermassive black hole called Sagittarius A* that is evolving on the timescale of mere minutes. This talk will present the methods and procedures used to produce the first image of Sagittarius A* as well as discuss future directions we are taking to map its evolving environment. It has been theorized for decades that a black hole will leave a “shadow” on a background of hot gas. However, due to its small size, traditional imaging approaches require an Earth-sized radio telescope. In this talk, I discuss techniques we have developed to photograph the Sagittarius A* black hole using the Event Horizon Telescope, a network of telescopes scattered across the globe. Imaging Sagittarius A* proved especially challenging due to the time-variability that had to be accounted for. Although we have learned a lot from these images already, remaining scientific questions motivate us to improve this computational telescope to recover these dynamics. This talk will then discuss how we are developing learning-based techniques that will allow us to extract the evolving structure of black holes in the future. In particular, we discuss how we can leverage the commonality in their evolving structure to recover a movie by inferring a shared image generator with a low-dimensional latent space. We then introduce Orbital Black Hole Tomography, which integrates known physics with a neural representation to map evolving flaring emission around the black hole in 3D for the first time.
Dr. Katherine L. (Katie) Bouman is an assistant professor in the Computing and Mathematical Sciences, Electrical Engineering, and Astronomy Departments at the California Institute of Technology. Her work combines ideas from signal processing, computer vision, machine learning, and physics to find and exploit hidden signals for scientific discovery. Before joining Caltech, she was a postdoctoral fellow in the Harvard-Smithsonian Center for Astrophysics. She received her Ph.D. in the Computer Science and Artificial Intelligence Laboratory (CSAIL) at MIT in EECS, and her bachelor’s degree in Electrical Engineering from the University of Michigan. She is a Rosenberg Scholar, Heritage Medical Research Institute Investigator, recipient of the Royal Photographic Society Progress Medal, Electronic Imaging Scientist of the Year Award, University of Michigan Outstanding Recent Alumni Award, and co-recipient of the Breakthrough Prize in Fundamental Physics. As part of the Event Horizon Telescope Collaboration, she is co-lead of the Imaging Working Group and acted as coordinator for papers concerning the first imaging of the M87* and Sagittarius A* black holes.
Complex-Valued Image Recovery from Multiple Fourier Measurements
Prof. Anne Gelb, Dartmouth
Because spotlight mode airborne synthetic aperture radar (SAR) is capable of all weather day-or-night imaging, it is a widely used imaging technology for surveillance and mapping. SAR phase history data can also be collected for the same scene at various elevation and azimuth angles. It is often imperative to obtain practically artifact- and noise-free SAR images on which practitioners can rely. While myriad computational methods have been developed to recover SAR imagery, difficult challenges remain. This talk presents a new recovery algorithm using an empirical Bayesian approach to address some of these challenges. Specifically, the method exploits the shared sparse feature space between multiple Fourier measurements (as a proxy for SAR phase history data) to guarantee robustness, accuracy, and efficiency, while simultaneously ensuring that phase information is not lost in the recovery process. The method is also designed to provide uncertainty quantification.
Dr. Anne Gelb is the John G. Kemeny Parents Professor of Mathematics at Dartmouth College. Her work focuses on high order methods for signal and image restoration, classification, and change detection for real and complex signals using temporal sequences of collected data. There are a wide variety of applications for her research including speech recognition, medical monitoring, credit card fraud detection, automated target recognition, and video surveillance. A common assumption made in these applications is that the underlying signal or image is sparse in some domain. While detecting such changes from direct data (e.g. images already formed) has been well studied, Professor Gelb’s focus is on applications such as magnetic resonance imaging (MRI), ultrasound, and synthetic aperture radar (SAR), where the temporal sequence of data are acquired indirectly. In particular, Professor Gelb develops algorithms that retain critical information for identification, such as edges, that is stored in the indirect data. Professor Gelb is currently investigating how to use these techniques in a Bayesian setting so that the uncertainty of the solutions may also be quantified, and is interested in applying these techniques for purposes of sensing, modeling, and data assimilation for sea ice prediction. Her research is funded in part by the Air Force Office of Scientific Research, the Office of Naval Research, the National Science Foundation, and the National Institutes of Health, and she regularly collaborates with scientists at the Wright-Patterson Air Force Research Lab and the Cold Regions Research and Engineering Laboratory (CRREL).
Massive Beam-Bandwidth Product Phased Arrays as RF Imaging Systems
Prof. Dennis Prather, University of Delaware

This presentation introduces a new class of phased array systems that offer a massive beam bandwidth product, of greater than 1THz, with element level and full-rank instantaneous beamforming. Such a capability enables true beamspace implementations of space-time adaptive processing (STAP), which can reduce the computational dependence for array optimization by orders of magnitude and beamspace synthetic aperture radar with a near real-time imaging capability. These new modalities stand on the shoulders of previous accomplishments that include real-time, video-rate imaging of passive RF scenes, which has enabled stand-off security screening when used in combination with active polarimetric imaging to uniquely identify certain objects of interest. In addition, these systems have been used to image active signals, i.e., emitters, in the electromagnetic environment and, when used in combination with engineered dispersive systems, can be used to provide a real-time visual rendering of k-space, i.e., co-location of instantaneous frequency measurement (IFM) and angle-of-arrival (AoA). The system uses an optical up-conversion technique that offers instant, full-rank beamforming with a computational dependency of O(1) and a latency limited only by the speed of light. This presentation will present the design, fabrication, and demonstration of several representative systems for both active and passive RF imaging along with future applications in STAP and SAR.
Professor Dennis Prather began his professional career by joining the US Navy in 1982, as an E-1, where he served for more than 38 years and recently retired as a CAPT (O-6) Engineering Duty Officer. After his initial tour of active duty, he attended the University of Maryland and received the BSEE, MSEE, and PhD degrees in 1989, 1993, and 1997, respectively. During his graduate study, he worked as a senior research engineer for the Army Research Laboratory, where he performed research on both optical devices and architectures for information processing. In 1997 he joined the Department of Electrical and Computer Engineering at the University of Delaware, where he is currently the College of Engineering Alumni Distinguished Professor. His research focuses on both the theoretical and experimental aspects of RF-photonic devices and their integration into operational systems for imaging, communications and Radar. To achieve this, his lab designs and develops fabrication/integration processes necessary for the demonstration of state-of-the-art RF-photonic devices such as: ultra-high bandwidth modulators, silicon photonic RF sources, photonic crystal chip-scale routers, meta-material antennas, and integrated RF-Photonic phased array antennas.
Professor Prather is currently an Endowed Professor of Electrical Engineering, he is a Fellow of the IEEE, Fellow of the Society of Photo-Instrumentation Engineers (SPIE), Fellow of the Optical Society of America (OSA) and Fellow of the National Academy of Inventors. He has authored or co-authored over 650 scientific papers, holds over 40 patents, and has written 16 books/book-chapters.
Covariant Wave Theory Foundations of Omega-K Synthetic Aperture Radar
Prof. Christopher Barnes, Georgia Institute of Technology
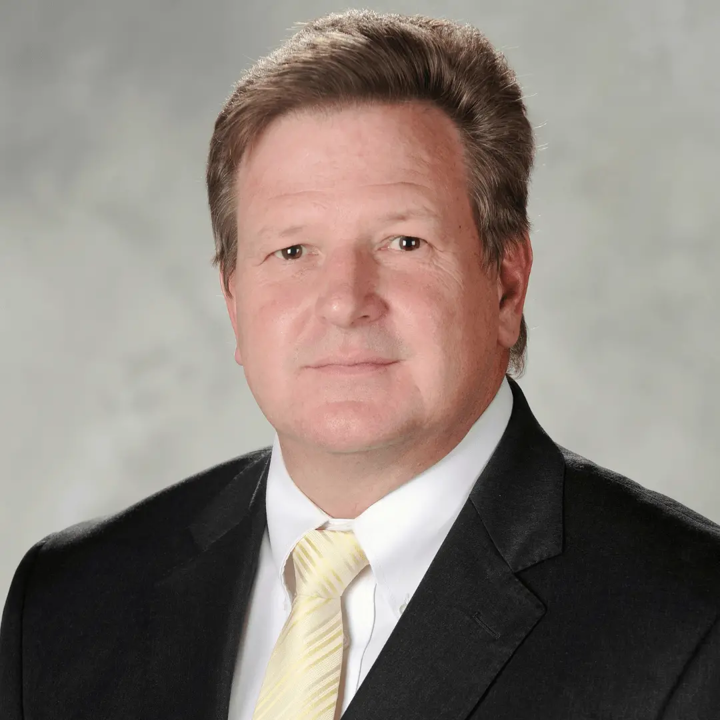
Advances in signal processing for aperture synthesis with omega-k signal processing that utilize a covariant change of wavenumber variables related to the quantum field theory work of P. A. M. Dirac are overviewed in this keynote address. These omega-k enhancements and capabilities rely on the availability of element data channels of a digital array radar (DAR). Since a DAR provides multiple spatial array samples, a baseline algorithm (usable without aperture synthesis) empowers single-pulse imaging at short range with a baseline (nonsynthesis) resolution. At longer range, the single-pulse baseline method also provides means for high-density digital beamforming-on-receive (HD-DBF). The single-pulse baseline method can be viewed as an all-range digital beamformer that is not constrained by a plane-wave assumption. The single-pulse omega-k method produces the same results achieved by time-space domain spherical backpropagation, but at greatly reduced computational complexity. Spherical wavefield inversion is accomplished at all ranges with efficient omega-k domain processing. With use of Dirac’s covariant frequency-wavenumber domain descriptions of free-space and scattered electromagnetic fields, the avoidance of plane-wave signal model approximations empowers a capability to coherently integrate multiple single-pulse data products (images and HD-DBF) for aperture synthesis. If relative movement increases angular dwell or reduces the range between sensor and scene on a pulse-to-pulse basis, then cross-range resolutions progressively improve as single-pulse images are coherently fused in the pixel domain. This keynote address provides an overview of the history of these wave theories, covariant methods, and application to SAR-with-DAR systems.
Dr. Christopher F. Barnes is an associate professor in the School of Electrical and Computer Engineering (ECE) at the Georgia Institute of Technology. Dr. Barnes received his Ph.D. degree from Brigham Young University in 1989, after which he joined the Georgia Tech Research Institute (GTRI) as research faculty. He transferred to the School of ECE as an associate professor in 2002, and retains GTRI adjunct status as a principal research engineer. Dr. Barnes has 27 years of experience in basic and applied research and has published approximately 140 papers and holds one patent. He is recognized internationally for his expertise in synthetic aperture radar (SAR) analysis and SAR image formation processing. Dr. Barnes has twenty years of experience teaching SAR at the professional education and graduate student level. He has invented radar imaging image formation processing methods capable of three-dimensional coherently fused SAR imaging in remote sensing applications. This advanced radar signal processing method has applications in sonar, medical imaging and seismology. He has provided subject matter expertise in many research and development oversight activities of ground based and ship based radar signal processing and radar software engineering programs.
Ptychographic Synthetic Aperture Imaging With Intensity-Only Measurements
Prof. Guoan Zheng, University of Connecticut
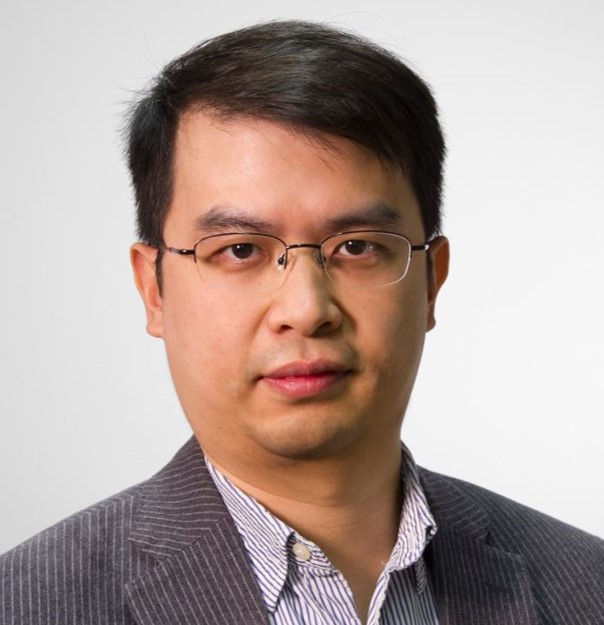
Ptychography has emerged as a transformative coherent diffraction imaging technique for both fundamental and applied sciences. Here we discuss a coded ptychography technique that achieves an imaging throughput order of magnitude greater than conventional microscopy approaches. In this platform, we translate the samples across the disorder-engineered surfaces for lensless diffraction data acquisition. The engineered surface can be made by smearing a monolayer of blood on top of the image sensor. The entire system can be built using a modified Blu-ray player, where the 405 nm laser from the optical pickup head can be used as a coherent light source for sample illumination. By tracking the phase wraps of the recovered images, we report the direct observation of bacterial growth in a 1-s interval at a 120 mm2 area, with a phase sensitivity like that obtained from interferometric measurements. We also characterize cell growth via longitudinal dry mass measurement and perform rapid bacterial detection at low concentrations. The combination of high phase sensitivity, high spatiotemporal resolution, and ultra-large field of view is unique among existing microscopy techniques. We will also discuss several extensions of the coded ptychography technique, including synthetic aperture ptychography, polarimetric coded ptychography, and depth-multiplexed ptychographic microscopy, thereby expanding its applicability in different fields.
Dr. Guoan Zheng received his B.S. degree from Zhejiang University in 2007 and the Ph.D. degree from Caltech in 2013. Upon completing his doctorate, he joined the University of Connecticut (UConn) as an Assistant Professor. Currently, Dr. Zheng is the United Technologies Corporation Associate Professor at UConn, with a joint appointment in both the Biomedical Engineering and Electrical Engineering Departments. Dr. Zheng’s research is dedicated to creating innovative imaging tools that address measurement challenges in biology and medicine. He has authored over 100 papers with more than 9700 citations. The Fourier ptychography approach he developed with his colleagues has been incorporated as a sub-chapter in the renowned textbook, “Introduction to Fourier Optics (4th edition)” by Goodman. Dr. Zheng also serves as the Senior Editor of PhotoniX, Associate Editor of Biomedical Optics Express, Associate Editor of Frontiers of Photonics, and the co-chair of the new conference, Computational Optical Imaging and Artificial Intelligence in Biomedical Sciences, in SPIE Photonics West.
High Spatiotemporal Resolution Interferometric Quantitative Phase Microscopy
Prof. Renjie Zhou, Chinese University of Hong Kong
Quantitative phase microscopy (QPM) is a holographic imaging technique which has found many important applications in biomedical imaging and material metrology. We have recently developed several high spatiotemporal resolution interferometric QPM techniques for high throughput cell imaging and nanometrology. I will first introduce our development of high spatiotemporal resolution synthetic aperture phase microscopy that can image and quantify millisecond-level fluctuations in living cells. I will further show our work on high-speed tomographic phase microscopy (TPM) and its applications on characterization of 3D-printed structures. After that, I will present our recent work on using deep learning to solve 3D inverse scattering problem and achieve single-frame TPM with an unprecedented speed of >10,000 volumes/second on imaging living cells. Finally, I will present our results on pushing the phase sensitivity to picometer level and profiling precision to sub-angstrom level, thus allowing us to probe subtle thickness differences in atomic layer structures.
Dr. Renjie Zhou is an Associate Professor of the Department of Biomedical Engineering and Assistant Dean (research) of Faculty of Engineering at the Chinese University of Hong Kong (CUHK), where he directs the Laser Metrology and Biomedicine Laboratory (LAMB). He received a PhD in Electrical and Computer Engineering from the University of Illinois at Urbana-Champaign in 2014 and undertook postdoctoral training at MIT between 2014-2017. His research interest is in developing optical precision instruments with applications in bioimaging and material metrology. He has published over 100 journal and conference papers and filed over 10 US/China patents with several licensed to industry. He is currently serving on the editorial boards of JOSA A, IEEE Photonics Technology Letters, and International Journal of Extreme Manufacturing. He is a Senior Member of Optica and SPIE.
FAIR Climate Services: Importance of Standards for UN Climate Policy Frames
Dr. Nils Hempelmann, Open Geospatial Consortium
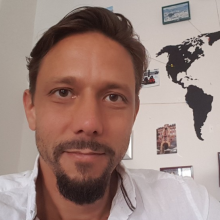
Over the last three decades, various policy initiatives have strived to limit the impacts of global warming, reverse land degradation, stop the loss of biodiversity, protect finite natural resources, and reduce risks associated with environmental disasters. Tracking the progress made by these initiatives is a complex task. It requires integrated work across multiple research domains, the deployment of data and technologies – including sophisticated observation instruments and networks, and large scale coordinated modeling experiments. Globally interconnected data and analytical infrastructures based on open standards are essential to the success of this work, because they enable the translation of raw data into reliable, actionable information. This is especially important in the UN climate policy conventions like UNFCCC, UNCCD or CBD, where standards are essential to ensure global comparability across nations delivering spatial information. To improve the efficiency of climate information generation and reporting, standardisation of the digital infrastructures, processing applications and reporting indicators are essential ingredients in the recipe towards climate change resilience. The data and digital infrastructures needs to follow FAIR principles, where FAIR stands for findable, accessable, interoperable and reusable. How FAIR principles can be applied in Climate Services and how important they are to underpin policy frames will be rolled out and discussed in this contribution. The concept presented can be seen as FAIR Climate Service.
Dr. Nils Hempelmann is responsible for planning and managing OGC Collaborative Solutions and Innovation Program initiatives. Mr. Hempelmann holds a PhD in Geography and provides a combination of an extensive scientific background in climate change data assessment and sustainable development as well as software engineering for scientific data processing and information delivery. Furthermore, he gained deep insights of the United Nations policy mechanisms e.g. as a member of the German UNCCD delegation as well as a UNFCCC observer. He contributes to OGC with his almost 20 years of research, scientific software development and climate service consultation experiences from international project contributions in research environments as well as consultation of international cooperation organizations.
Automotive Synthetic Aperture Radar; State-of-the-Art and Future Perspective
Prof. Marco Manzoni, Politecnico di Milano
In recent years, we have seen growing scientific attention toward the Automotive Synthetic Aperture Radar (SAR) concept. This technique allows to synthesize a long aperture by exploiting the vehicle’s motion, thus reaching very high performances in terms of spatial resolution and Signal-to-Noise-Ratio (SNR). Indeed, the achieved performances are comparable to or even greater than those reached using massive Multiple-Input-Multiple-Output (MIMO) imaging arrays that are way more expensive and complex. This talk dives into this technology’s state of the art, its nuances, and all the tips and tricks to make it work in a practical scenario. Several factors must be considered to make this technology work properly: different acquisition geometries must be considered, a fast and robust focusing algorithm must be designed, and a method to refine the vehicle’s trajectory must be engineered to achieve the aforementioned performances.
The talk also tries to look at the future of this technology. Several vehicles could interact in a multistatic approach: in this scenario, one vehicle transmits a waveform and record the echo, but at the same time, another one records it. By sharing the echo information between them in a networked scenario, better performances can be achieved regarding coverage, resolution, and SNR. However, this innovative approach brings challenges, including time synchronization between vehicles and sidelobes induced by spectral gaps in the multistatic images. All these aspects will be broadly discussed during the talk.
Finally, another possible path that the future of this technology could take is the one dictated by the Joint Communication & Sensing (JC&S), in which the transmitted waveforms also carry information from one vehicle to another entity (V2X). The talk discusses the perspective, challenges, and possible methods to make this future possible.
Dr. Marco Manzoni was born in Lecco, Italy, in 1994. He received the Ms.C. Degree (Cum Laude) in Telecommunications Engineering in 2018 from Politecnico di Milano and the Ph.D. in Information Technology (Cum Laude) from the same institution in 2022. He is currently an Assistant Professor at Politecnico di Milano. His research interests span signal processing for radar remote sensing, automotive Synthetic Aperture Radar (SAR), Joint Communication and sensing (JC&S), Drone-based SAR, autofocusing algorithms for SAR imaging, SAR Interferometry (InSAR) and SAR calibration. He is the recipient of the 2022 Dimitris N. Chorafas Award, co-recipient of the Best Paper Award for the 2022 Mediterranean Microwave Symposium, and the PIERS 2023 Young Scientist Award winner. He served as Technical Session Chair at IEEE RadarConf’23 and is currently chair of the IEEE Automotive SAR Working Group, developing standards for vehicle-based SAR systems.
Phaseless Synthetic Aperture Radar Imaging: Advances, Challenges and Future Prospects
Dr. Bariscan Yonel, Rensselaer Polytechnic Institute

Phaseless synthetic aperture radar (SAR) is a novel imaging modality that can have profound implications on the development of future remote sensing systems due to the advantages in hardware cost and complexity, robustness to phase errors, and operability at high frequencies. Despite the ill-posed nature of the generic phase retrieval problem, significant strides have been made in the fields of optics and data science to establish theoretical guarantees for phaseless imaging using novel optimization methods and properties that are commonly established for statistical measurement models. However, synthetic aperture formation in the lack of phase information is a major challenge for conventional SAR systems as they lack the illumination diversity and spatial incoherence properties to enable utilizing such existing, state-of-the-art algorithmic procedures and the associated theoretical guarantees. In this talk, we present the recent advances in the phase retrieval literature, and the developments towards the realization of phaseless SAR imaging by virtue of illumination diversity that can facilitate accessing the state-of-the-art theory, methods, and algorithms. We ultimately motivate utilizing multi-static configurations for the illumination of a scene of interest, which can be used to generate superpositions that imitate spatially incoherent fields with diversity within and across pulses using stochastically modulated waveforms. We overview the fundamental considerations towards the realization of such a phaseless multi-static SAR imaging system, and outline the challenges, ongoing efforts, and the potential of this technology for large-scale distributed sensing.
Dr. Bariscan Yonel (Member, IEEE) received his B.Sc. degree in electrical engineering from Koc University, Istanbul, Turkey, in June 2015, and the Ph.D. degree in electrical engineering from Rensselaer Polytechnic Institute (RPI) Troy, NY in December 2020. He has been a postdoctoral research associate at RPI with the Computational Imaging Group since May 2021. His research interests span applications and performance analysis of machine learning, compressed sensing, and optimization methods for inverse problems in imaging and remote sensing. He currently works towards theoretical guarantees and practical limitations for solving quadratic systems of equations using low-rank matrix recovery theory and computationally efficient non-convex algorithms, with applications to high dimensional inference, wave-based imaging, and array signal processing.