Introduction to Synthetic Aperture Radar
Monday, May 20
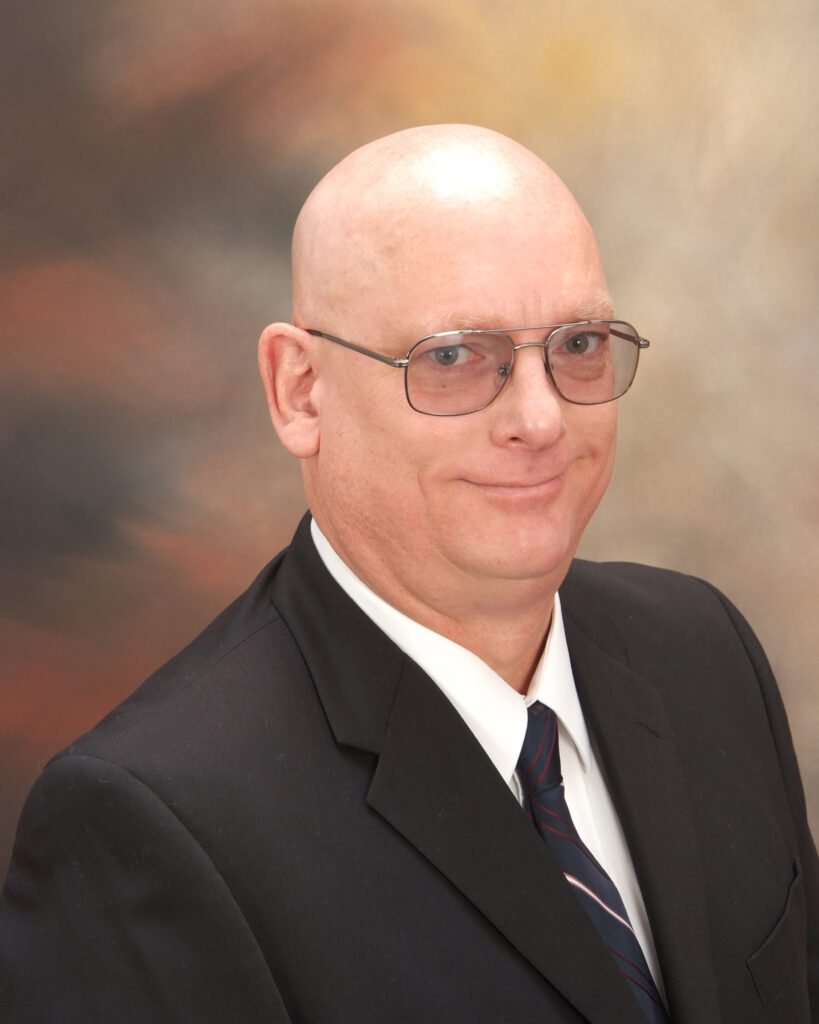
Synthetic Aperture Radar (SAR) is a radar imaging mode that maps radar reflectivity of the ground. This is an important earth resource monitoring and analysis tool in the civilian and government communities, and an important intelligence, surveillance, and reconnaissance (ISR) tool for the military and intelligence communities. The tutorial proposed herein is intended to provide an introduction to the physical concepts, processing, performance, features, and exploitation modes that make SAR work, and make it useful. Although mathematics will be shown in some parts of the presentation, the lecture will focus on the qualitative significance of the mathematics rather than dry derivations. Liberal use of example SAR images and other data products will be used to illustrate the concepts discussed. The presentation will be given as four distinct modules, each based on (but enhanced from) presentations developed and given by the presenter in numerous non-public forums to government, military, industry, and academic groups.
Presenter: Dr. Armin Doerry is a Distinguished Member of Technical Staff in the ISR Mission Engineering Department of Sandia National Laboratories. He holds a Ph.D. in Electrical Engineering from the University of New Mexico. He has worked in numerous aspects of Synthetic Aperture Radar and other radar systems’ analysis, design, and fabrication since 1987, and continues to do so today. He has taught Radar Signal Processing classes (and related topics) as an adjunct professor at the University of New Mexico, and has taught numerous seminars on SAR and other radar topics to government, military, industry, and academic groups.
Introduction to Inverse Synthetic Aperture Radar
Monday, May 20

Inverse synthetic aperture radar images are generated from radar scattered data collected from a rotating target withing the radar’s beam. It is a version of synthetic aperture radar and has been used extensively over the last three decades to image dynamic targets such as ships, aircrafts, space objects, and ground moving vehicles. High resolution radar images with fine cross-range resolution traditionally require a large array with lots of antenna elements, and precise phase synchronization across the multiple channel data. ISAR offers a cheaper alternative for obtaining fine cross-range resolution by requiring only single-channel broadband data by leveraging the wide aspect presented by a rotating target to the radar. The key challenges associated with ISAR imaging are the removal/compensation of the relative translational motion, and higher order rotational motion of the target. Recently, ISAR imaging has been extended to automotive targets for automatic target recognition and classification using modern machine learning, and deep learning algorithms The objective of the tutorial is to provide an introduction to ISAR concepts, present algorithms required for generating ISAR images, discussion on the ISAR motion compensation algorithms, as well as variants of ISAR along higher dimensions, followed by discussions on electromagnetic modeling of dynamic targets to simulate ISAR images and measurement data collection with Texas Instruments millimeter wave radar.
Presenter: Shobha Sundar Ram did her Bachelor of Technology in ECE from the University of Madras, India in 2004 and then her Master of Science and Ph.D. in electrical engineering from the University of Texas at Austin, USA in 2006 and 2009 respectively. She worked as a research and development electrical engineer at Baker Hughes Inc. USA from 2009 to 2013. She joined Indraprastha Institute of Information Technology as an Assistant Professor in 2013 where she is currently an Associate Professor in the Dept. of Electronics and Communications Engineering. She served as the Head of the Dept. of Electronics and Communications Engineering between 2019 and 2021 and is currently serving as the Dean of Student Affairs. She is primarily engaged in research and education principally in the areas of radar signal processing and electromagnetic sensor design and modeling. She is a Senior Member of IEEE, an Associate Editor for the IEEE Transactions on Aerospace and Electronics Systems, a member of the IEEE Radar Systems Panel for AESS Society, a member of the Synthetic Aperture Technical Working Group for the IEEE Signal Processing Society, Secretary for Synthetic Aperture Radar Standards Committee, Guest Editor for IET Radar Sonar and Navigations and Topics Editor for Frontiers on Signal Processing. She is currently serving the Technical Program Committee Co-chair of IEEE MAPCON 2024, Hyderabad, India. She has served as a Technical Program Committee member for numerous national and international conferences. She has won student paper awards for the IEEE Radar Conference in 2008 and 2009and won best paper awards for co-authored papers at the International Workshop on Non-intrusive Load Monitoring 2016, IEEE International Microwave and RF Conference 2019 and IEEE Radar Conference 2020. She has won the 2022 Qualcomm Innovation Fellowship India award and the 2023 Associate Editor Recognition Award for IEEE Transactions on Aerospace and Electronics Systems.
Phase Retrieval in Synthetic Aperture Imaging and Sensing
Monday, May 20
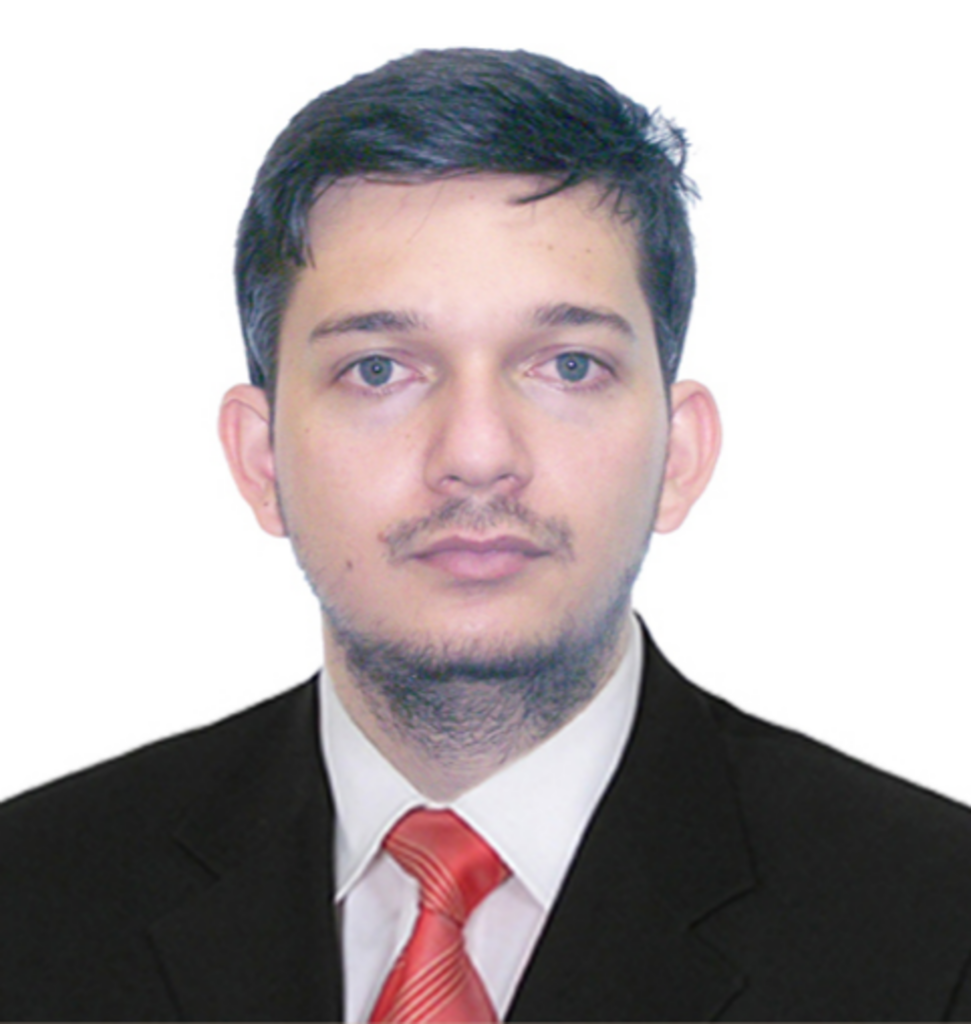
Synthetic aperture (SA) systems generate a larger aperture with greater spatial/temporal resolution than is inherently possible from the physical dimensions of a single sensor alone. These apertures are found in various signal processing applications such as optics, radar, remote sensing, microscopy, acoustics, and tomography. The SA processing often involves phase retrieval (PR), wherein a complex signal is to be recovered from the phaseless data. In general, both convex and nonconvex approaches have been suggested to solve the generic PR problem. However, these techniques are not readily applicable to various SA problems. In this tutorial, we provide a deep dive into the recent advances in PR for contemporary synthetic aperture imaging and sensing applications. Depending on the linear propagation model, diverse and scattered applications can be grouped into four main categories: Fourier PR, coded illumination, coded detection, and random. We cover the respective theories, algorithms, and use cases, including applications of machine learning in this area. This tutorial also aims to foster interaction between various SA disciplines thereby leading to a better understanding of the PT problems.
Presenter 1: Samuel Pinilla (S’17) received the B.S. degree (cum laude) in Computer Science in 2014, the B.S. degree in Mathematics, and the M.S degree in Mathematics from Universidad Industrial de Santander, Bucaramanga, Colombia in 2016 and 2017, respectively. His Ph.D. degree from the Department of the Electrical and Computer Engineering, Universidad Industrial de Santander, Bucaramanga, Colombia. He is a senior data scientist at the Science and Technology Facilities Council, United Kingdom. In the past, Dr. Pinilla held Visiting Postdoctoral Researcher positions at Tampere University 2020-2021 and worked as a fellow research associate at The University of Manchester 2021-2022. His research interests focus on the areas of high-dimensional structured signal processing, machine learning, scalable AI, and (non)convex optimization methods. Dr. Pinilla is the recipient of the Eloy Valenzuela Prize for his doctoral studies, the International Conference on Acoustics, Speech and Signal Processing top 3% Paper Recognition in 2023.
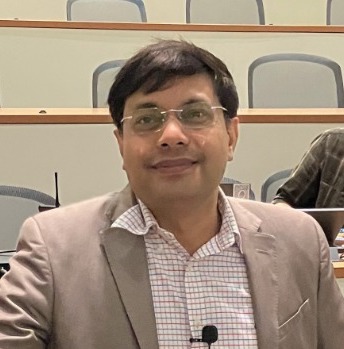
Presenter 2: Kumar Vijay Mishra (S’08-M’15-SM’18) obtained a Ph.D. in electrical engineering and M.S. in mathematics from The University of Iowa in 2015, and M.S. in electrical engineering from Colorado State University in 2012, while working on NASA’s Global Precipitation Mission Ground Validation (GPM-GV) weather radars. He received his B. Tech. summa cum laude (Gold Medal, Honors) in electronics and communication engineering from the National Institute of Technology, Hamirpur (NITH), India in 2003. He is currently Senior Fellow at the United States Army Research Laboratory (ARL), Adelphi; Technical Adviser to Singapore-based automotive radar start-up Hertzwell and Boston-based imaging radar startup Aura Intelligent Systems; and honorary Research Fellow at SnT – Interdisciplinary Centre for Security, Reliability and Trust, University of Luxembourg. Previously, he had research appointments at Electronics and Radar Development Establishment (LRDE), Defence Research and Development Organisation (DRDO) Bengaluru; IIHR – Hydroscience & Engineering, Iowa City, IA; Mitsubishi Electric Research Labs, Cambridge, MA; Qualcomm, San Jose; and Technion – Israel Institute of Technology.
Dr. Mishra is the Distinguished Lecturer of the IEEE Communications Society (2023-2024), IEEE Aerospace and Electronic Systems Society (AESS) (2023-2024), IEEE Vehicular Technology Society (2023-2024), and IEEE Future Networks Initiative (2022). He is the recipient of the IET Premium Best Paper Prize (2021), U. S. National Academies Harry Diamond Distinguished Fellowship (2018-2021), American Geophysical Union Editors’ Citation for Excellence (2019), Royal Meteorological Society Quarterly Journal Editor’s Prize (2017), Viterbi Postdoctoral Fellowship (2015, 2016), Lady Davis Postdoctoral Fellowship (2017), DRDO LRDE Scientist of the Year Award (2006), NITH Director’s Gold Medal (2003), and NITH Best Student Award (2003). He has received Best Paper Awards at IEEE MLSP 2019 and IEEE ACES Symposium 2019.
Dr. Mishra is Chair (2023-present) of the Synthetic Apertures Technical Working Group of the IEEE Signal Processing Society (SPS) and Vice-Chair (2021-present) of the IEEE Synthetic Aperture Standards Committee, which is the first SPS standards committee. He is the Chair (2023-2026) of the International Union of Radio Science (URSI) Commission C. He has been an elected member of three technical committees of IEEE SPS: SPCOM, SAM, and ASPS, and IEEE AESS Radar Systems Panel. Since 2020, he has been Associate Editor of IEEE Transactions on Aerospace and Electronic Systems, where he was awarded Outstanding Editor recognition in 2021. He has been a lead/guest editor of several special issues in journals such as IEEE Signal Processing Magazine, IEEE Journal of Selected Topics in Signal Processing, and IEEE Journal on Selected Areas in Communications. He is the lead co-editor of three books on radar: Signal Processing for Joint Radar-Communications (Wiley-IEEE Press), Next-Generation Cognitive Radar Systems (IET Press Radar, Electromagnetics & Signal Processing Technologies Series), and Advances in Weather Radar Volumes 1, 2 & 3 (IET Press Radar, Electromagnetics & Signal Processing Technologies Series). His research interests include radar systems, signal processing, remote sensing, and electromagnetics.
Understanding and Correcting Motion Artefacts in MRI
Monday, May 20

MRI scans are typically longer than physiological motion. If unaccounted for, motion can create major image artefacts and reduce diagnostic confidence. In this lecture, we will learn how motion artefacts are generated, some of the general solutions employed to deal with motion, and introduce a generalized formalism to deal with motion in MRI. Following, we will experiment with a code demo of generalized motion correction and study its behaviour in several scenarios.
Note: Attendess are invited to bring their laptop to this tutorial to participate in problem solving demonstrations
Presenter: Dr. Gastao Cruz did his PhD at King’s College London working with Drs. Claudia Prieto, David Atkinson, Tobias Schaeffter and Rene Botnar, working in motion correction for cardiac and abdominal MRI. During his post-doctoral work, his research developed motion correction methods for multi-property quantitative MRI. He his now an Assistant Professor at the University of Michigan, where he continues to advance quantitative MRI using state-of-the-art frameworks like MR Fingerprinting. He is a Junior Fellow of the ISMRM.
Synthetic Aperture Imaging with Neural Signal Representations
Monday, May 20
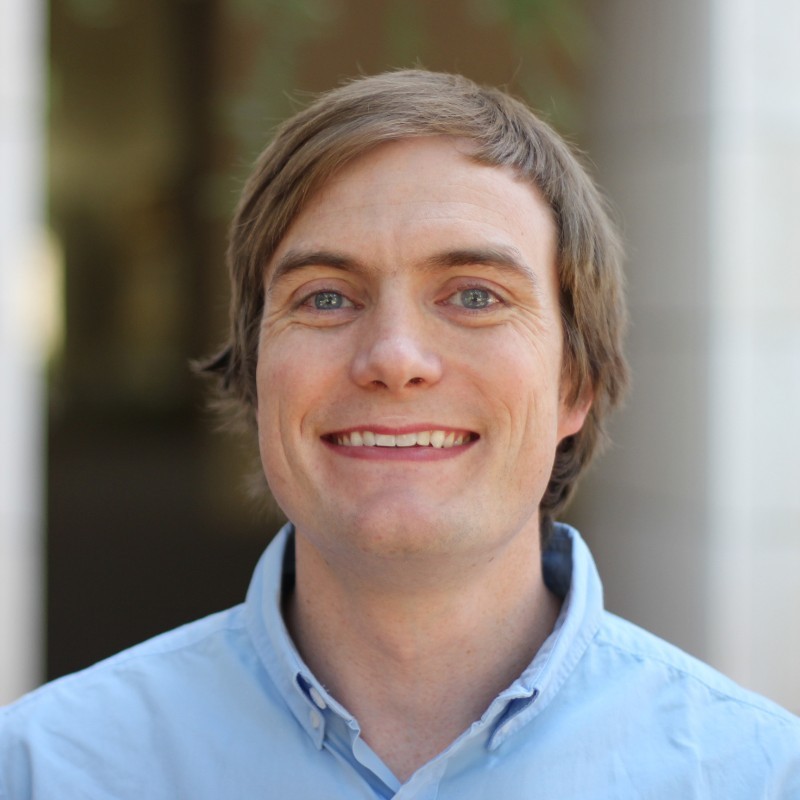
Synthetic aperture imaging is a powerful computational imaging technique wherein a collection of low resolution measurements are computationally integrated into a single high-resolution image. This fusion step has been traditionally accomplished with pixel-based scene representations. This tutorial provides an introduction to neural signal representations, an alternative signal representation that models signals as functions. It then describes how neural signal representations can be used to enhance the synthetic aperture reconstruction pipeline by automatically capturing and exploiting spatial, temporal, and spectral regularity.
Presenter: Chris Metzler is an Assistant Professor in the Department of Computer Science (and ECE by courtesy) at the University of Maryland, where he leads the UMD Intelligent Sensing Laboratory. His research develops new systems and algorithms for solving problems in computational imaging and sensing, machine learning, and wireless communications. His work has received multiple best paper awards; he recently received NSF CAREER and AFOSR Young Investigator Program awards; and he was an Intelligence Community Postdoctoral Research Fellow, an NSF Graduate Research Fellow, a DoD NDSEG Fellow, and a NASA Texas Space Grant Consortium Fellow.